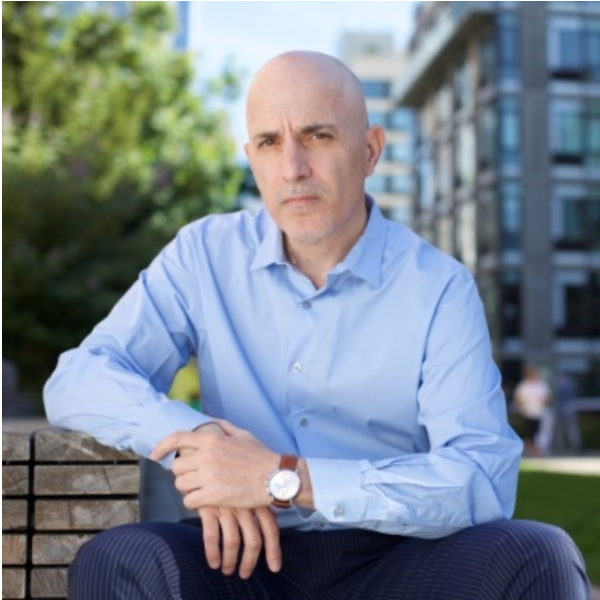
In the digital age, data is often described as the new oil, powering transformations across industries, from healthcare to finance, and beyond. As businesses and organizations grapple with an ever-increasing volume of data, the need for advanced tools to analyze, interpret, and derive actionable insights from this data has become paramount. Artificial Intelligence (AI) has emerged as a cornerstone technology in this endeavor, offering powerful capabilities to streamline processes, uncover patterns, and predict future trends. This article explores the top seven AI tools that are reshaping the landscape of data analysis and insights.
1. TensorFlow
Developed by Google Brain, TensorFlow is an open-source library for numerical computation and large-scale machine learning. TensorFlow bundles together a slew of machine learning and deep learning models and algorithms, making them useful for computing data flow graphs. The tool’s flexible architecture allows users to deploy computation to one or more CPUs or GPUs in a desktop, server, or mobile device with a single API. It is particularly powerful in training and inference of deep neural networks and is extensively used in both academia and industry for a range of tasks including speech recognition, computer vision, robotics, drug discovery, etc.
2. PyTorch
Facebook’s AI Research lab developed PyTorch, an open-source machine learning library based on the Torch library, for applications such as computer vision and natural language processing. Known for its flexibility and speed, PyTorch excels in projects that involve complex neural networks. PyTorch’s dynamic computational graphing, a key feature that allows changes to the network behavior on-the-fly, makes it highly suited for research and prototypical projects that require frequent adjustments.
3. IBM Watson
IBM Watson stands out in the AI landscape for its advanced data analysis capabilities, particularly in natural language processing. Watson can understand all forms of data, interact naturally with people, and learn and reason at scale. It’s capable of analyzing and interpreting all of your data, including unstructured text, images, audio, and video. One of Watson’s most notable applications is in healthcare, where it helps professionals make faster and more informed decisions by processing vast amounts of medical literature and patient data against established, evidence-based guidelines.
4. Google Cloud AI
Google Cloud AI is a suite of AI services designed to help organizations solve their most challenging problems using data and machine learning. The tool includes a variety of services such as AI Hub, AI Building Blocks, and AutoML. Google Cloud AI makes it easy to use machine learning models, whether they’re custom-built or pre-trained. You can use it for translation, natural language processing, and image analysis. Google Cloud AI is a useful tool for businesses that wish to expand their AI capabilities. By integrating with Google’s strong cloud infrastructure and data analytics features, businesses can take advantage of its power.
5. Microsoft Azure Machine Learning
Microsoft Azure Machine Learning is a cloud-based platform for training, deploying, and managing machine learning models. Azure ML is a platform that supports machine learning. It allows users to work with popular machine learning frameworks like PyTorch, TensorFlow, and scikit-learn. The platform also offers tools to help users prepare data, design and train models, and deploy them into production. Automated machine learning is also supported, which automates the selection and tuning of machine learning models. This makes it accessible to both experienced data scientists and developers without deep data science backgrounds.
6. Amazon SageMaker
Amazon SageMaker is a service that makes it easy for developers and data scientists to create, train, and launch machine learning models. It’s fully managed, so you don’t have to worry about infrastructure management. SageMaker removes the heavy lifting from each step of the machine learning process to make it easier to develop high-quality models. Noteworthy features include one-click model training, hyperparameter tuning, and easy deployment to a production-ready hosted environment. SageMaker’s broad integration with other AWS services enhances its utility, making it an excellent choice for developers looking to weave AI and ML into their existing applications.
7. RapidMiner
RapidMiner is a data science platform that provides an integrated environment for data preparation, machine learning, deep learning, text mining, and predictive analytics. It particularly suits those who prefer a graphical interface that enables them to build and visualize their data analysis workflows. RapidMiner supports all stages of the data science lifecycle, from preparation to model deployment, and offers enough flexibility for use in business applications, as well as research and educational purposes.
The advancement of AI tools has dramatically transformed the possibilities within data analysis and insights. The top seven AI tools discussed here each offer unique strengths, making them valuable assets in the data scientist’s toolkit. These tools can help you analyze data more efficiently and effectively, whether you’re a seasoned data scientist, a business analyst, or new to the field. It’s important to choose the right tool based on your needs. Keep sentences short and to the point, and use everyday language instead of jargon or acronyms. Use active voice and verbs instead of nouns to make the text clearer and easier to understand. Remember to put the most important information first to create a logical structure. As these technologies continue to evolve, they will play a pivotal role in driving innovation, optimizing operations, and enhancing decision-making processes across all sectors.